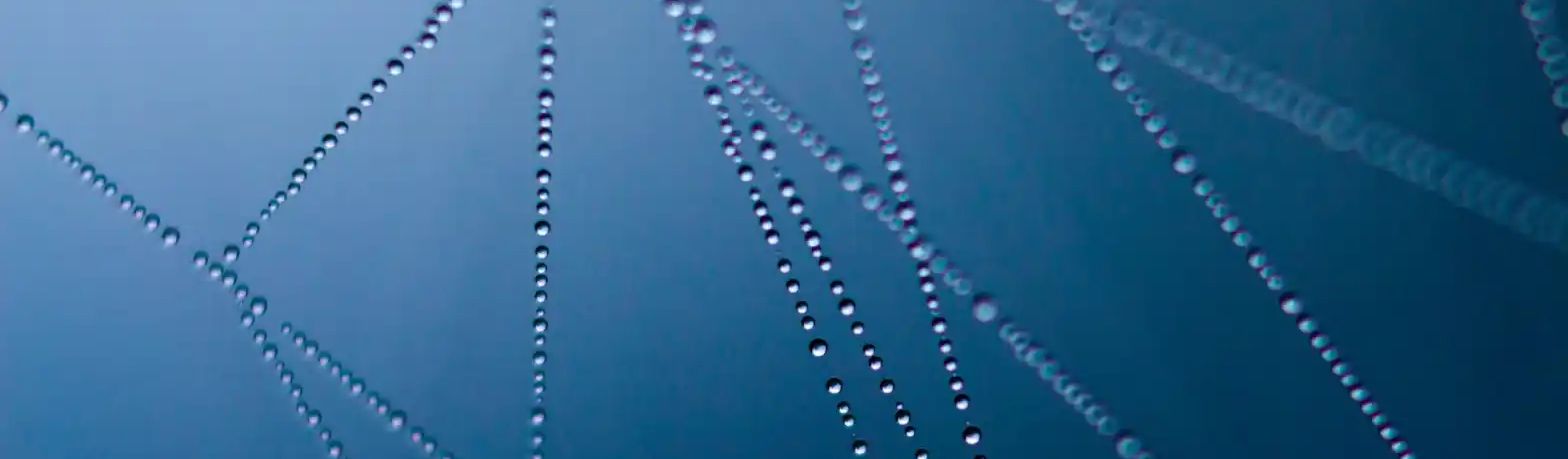
Lecture Series: Philosophy of Science and Machine Learning
Description
In an age where artificial intelligence (AI) is transforming science, it becomes increasingly important to reflect critically on the foundations, methodologies, and implications of these advancements. This lecture series will investigate fundamental issues in AI from the vantage point of philosophy of science, which includes topics such as the transparency and interpretability of AI within scientific research, or the impact of AI on scientific understanding and explanation.
This lecture series is a special edition of the AI Colloquium at TU Dortmund University, coorganized by the Lamarr Institute for Machine Learning and Artificial Intelligence, the Research Center Trustworthy Data Science and Security (RC Trust), and the Center for Data Science & Simulation at TU Dortmund University (DoDas). The Lecture Series is organized by the Emmy Noether Group „UDNN: Scientific Understanding and Deep Neural Networks”, and generously funded by the German Research Foundation (DFG; grant 508844757).
Time & Place
Thursdays, 4:15pm, room 303, 3rd floor, Joseph-von-Frauenhofer-Str. 25, 44227 Dortmund
Speakers
17.10.24 André Curtis Trudel (University of Cincinnati): „On finding what you’re (not) looking for: prospects and challenges for AI-driven discovery“
Abstract:
Recent high-profile scientific achievements by machine learning (ML) and especially deep learning (DL) systems have reinvigorated interest in ML for automated scientific discovery (e.g., Wang et al. 2023). Much of this work is motivated by the thought that DL methods might facilitate the efficient discovery of phenomena, hypotheses, or even models or theories more efficiently than traditional, theory-driven approaches to discovery. This talk considers some of the more specific obstacles to automated, DL-driven discovery in frontier science, focusing on gravitational-wave astrophysics (GWA) as a representative case study. In the first part of the talk, we argue that despite these efforts prospects for DL-driven discovery in GWA remain uncertain. In the second part, we advocate a shift in focus towards the ways DL can be used to augment or enhance existing discovery methods, and the epistemic virtues and vices associated with these uses. We argue that the primary epistemic virtue of many such uses is to decrease opportunity costs associated with investigating puzzling or anomalous signals, and that the right framework for evaluating these uses comes from philosophical work on pursuitworthiness.
28.11.24 Tim Räz (University of Bern) „The Concept of Memorization in Machine Learning“ (cancelled)
28.11.23 Leda Berio (RU Bochum): „Social interaction with artificial agents: when, how, why? Perspective taking and social scripts in HRI and interaction with AI“
When a robotic dog wags its tail, we do not hesitate to interpret it as a sign of happiness. We get upset when seeing little robot dinosaurs, barely more than sophisticated toys, mistreated. We hesitate to strike little bug-like robotic objects. Evidence shows, in other words, that our interactions with robots are laden with affect; and this is despite our full awareness that robots do not, ultimately, feel. What are the factors that influence these attributions? What aspects of design can influence the way we interact with artificial agents? I ague that considering interactions with artificial agents in terms of emotionally-loaded scripts can contribute to explaining our attribution of emotional states to social robots as well as our emotional reactions during interactions with them. Moreover, it helps us identify the normative components of such interactions. In this talk, I first explore the ways design features influence basic mechanisms of spontaneous perspective taking with social robots, presenting data that shows how visual appearance modulates these effects. Next, I propose that to explain more sophisticated mental state attribution, we should consider social interactions as activating scripts and schemata (Bicchieri and McNally, 2018) that come with expectations on how agents should behave and feel. Scripts contain information about expected emotional reactions, and their activation prescribe the interpretation of emotions in normative ways, as well as emotional attributions. In this sense, I suggest, when interacting with social robots, our behaviors and emotions, as well as our attributions, are highly normatively regulated. To conclude, I discuss how basic design features relate to the activation of scripts.
12.12.24 Stefan Buijsman (TU Delft): „The impact of epistemic dependence on AI for understanding“
The introduction of AI into our knowledge-gathering practices and decision making often means that we also become (somewhat) epistemically dependent on these systems. We rely on them to make it easier for us to know and understand phenomena. This can be highly beneficial, similar to how we use instruments in a lot of settings to more easily acquire knowledge and understanding. The specific nature of AI, primarily its opacity and often unexpected lapses in reliability, pose challenges however to beneficial epistemic dependence. In this talk I go over risks to our competence and responsibility as we increase our epistemic dependence on AI, and offer some suggestions on how we might deal with those.
23.01.25 Nina Poth (Radboud University Nijmegen): „AI in Tension Between Cognitive Science and Society“
In this talk, I examine the dual role of AI in both cognitive science and society. On one hand, significant ethical and theoretical concerns that call for caution in overstating the similarities between human-like intelligence and cutting-edge AI systems widely used today (such as AlexNet, GPT-4, etc.). On the other hand, cognitive scientists and philosophers view such deep neural networks as valuable tools for modelling and gaining insights into the human mind. These two perspectives stand in tension with one another that calls for a re-examination of the role of AI in cognitive science. While there are promising exploratory successes in specific domains such as perceptual categorisation, the contributions of deep neural networks to advancing our understanding of cognition are less revolutionary than often claimed and better put as a continuation of earlier revisions to computationalism, posing the worry that relative to the immense resources going in to building these models, the payoffs from theory lag significantly behind.
Participation
Participation is free, but places are limited. If you are interested in participating online, please register via the following form: https://forms.microsoft.com/r/W3whw0ac3B. If you would like to attend in person, please send an e-mail to udnn.ht@tu-dortmund.de.
We look forward to your participation and insightful discussions.
Kind regards,
The UDNN team (https://udnn.tu-dortmund.de/)
Main Organizers
Annika Schuster, Frauke Stoll, and Florian J. Boge