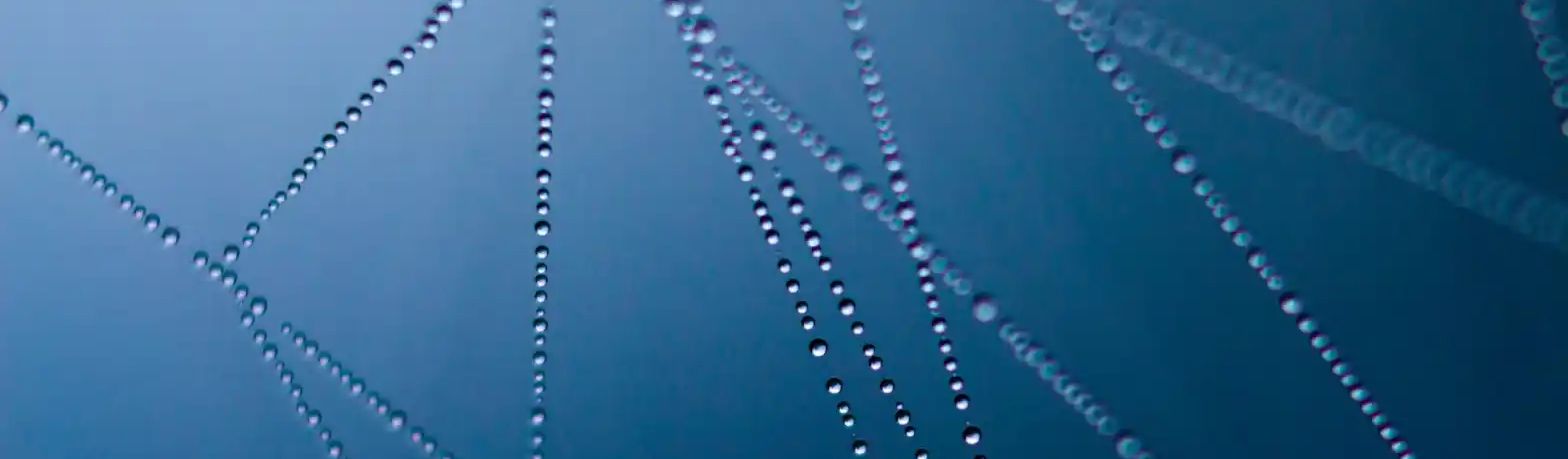
Workshop:
Epistemological Issues of Machine Learning in Science
Date: 27.–28.02.2024
Place: Chaudoire Pavillon, TU Dortmund University
Description
With impressive advances in Machine Learning (ML) and particularly Deep Learning, Artificial Intelligence is currently taking science by storm. This workshop brings together top scientists and philosophers working on fundamental issues connected to the use of Machine Learning in science. The workshop marks the launch of the DFG-funded Emmy Noether Group UDNN: Scientific Understanding and Deep Neural Networks, and is co-organized with the Lamarr Institute for Machine Learning and Artificial Intelligence and co-funded by the Department for Humanities and Theology at TU Dortmund University.
Topics include, but are not restricted to:
- The relation between prediction and discovery on the one hand, and explanation and understanding on the other, in fields of science that heavily rely on ML methods
- The key issues in identifying genuine discoveries and stable predictions by ML systems
- Core conceptions of “explanation” involved in the field of eXplainable AI (XAI), and their relation to philosophical theories of understanding and explanation
- Present limitations associated with ML’s predictive power and what may be needed to overcome them
- The connection between ML and traditional scientific means for prediction and discovery, such as theories, models, and experiments
- Our present understanding of ML itself and its limitations
Speakers
Life Sciences
- Jürgen Bajorath (University of Bonn)
- Axel Mosig (Ruhr University Bochum)
Machine Learning Theory
- M. Klopotek (University of Stuttgart)
- Marie-Jeanne Lesot (Sorbonne Université)
- David Watson (King’s College London)
Philosophy
- Katie Creel (Northeastern University)
- Brigitte Falkenburg (TU Dortmund)
- Konstantin Genin (University of Tübingen)
- Lena Kästner (University of Bayreuth)
- Henk de Regt (Radbout University Nijmegen)
- Eva Schmidt (TU Dortmund)
- Tom Sterkenburg (LMU Munich)
Physics / Astronomy
- Dominik Elsässer (TU Dortmund)
- Michael Krämer (RWTH Aachen)
- Mario Krenn (Max Planck Institute for the Science of Light)
- Wolfgang Rhode (TU Dortmund)
- Christian Zeitnitz (BU Wuppertal)
Registration is free but places are limited. To register, please send an E-mail to udnn.fk14@tu-dortmund.de until January 15, 2024 including your name, institution. A small number of attendees will be able to join the conference dinner on the 27th on a dutch-treat basis. If you want to join the dinner, please indicate this in your registration.
Schedule
Day 1 | Day 2 | ||
---|---|---|---|
09:00–09:15 | Arrival + Coffee | 09:00–09:15 | Arrival + Coffee |
09:15-09:20 | Opening (FJB) | 09:15 – 10:00 | From the fair distribution of predictions to the fair distribution of
social goods: evaluating the impact of fair machine learning on long-term
unemployment Konstantin Genin, Tübingen |
09:20-10:05 | Can machines acquire scientific understanding? Henk de Regt, Nijmegen |
10:00 – 10:45 | Explainable AI and trustworthy AI: a relation to discuss Marie-Jeanne Lesot, Paris |
10:05–10:50 | Richness revisited: clustering and PAC learnability David Watson, London |
10:45 – 11:00 | Coffee |
10:50–11:05 | Coffee | 11:00 – 11:45 | Towards an artificial muse for new ideas in science Mario Krenn, Erlangen |
11:05–11:50 | Occam’s razor in machine learning Tom Sterkenburg, Munich |
11:45 – 12:15 | Navigating the black box: Understanding particle
physics with deep neural networks and explainable artifical intelligence
Frauke Stoll, Dortmund |
11:50–12:20 | A new pathway: From objectual to explanatory understanding with
AlphaFold2 Annika Schuster, Dortmund |
12:15–13:15 | Lunch |
12:20–13:20 | Lunch | 13:15 – 14:00 | What can we learn from and through machine learning if the physics of
many-body systems is behind it? Miriam Klopotek, Stuttgart |
13:20–14:05 | Is knowledge forever? An astronomical perspective Dominik Elsässer, Dortmund |
14:00 – 14:45 | Stakes and understanding the decisions of artificial intelligent
systems Eva Schmidt, Dortmund |
14:05–14:50 | ML-driven knowledge gain in physics Wolfgang Rhode, Dortmund |
14:45 – 15:30 | A hypothesis-centric perspective on machine learning in biomedicine
Axel Mosig, Bochum |
14:50–15:35 | Data, theories, and machine learning in astroparticle physics Brigitte Falkenburg, Dortmund |
15:30 – 15:45 | Coffee |
15:35–15:50 | Coffee | 15:45 – 16:30 | (to be announced) Kathleen Creel, Boston |
15:50–16:35 | Opacity as a stepping stone Lena Kästner, Bayreuth |
16:30 – 17:15 | Deep learning for scientific discovery and the theory-freedom-robustness
trade-off Florian Boge, Michael Krämer, Christian Zeitnitz, Dortmund / Aachen / Wuppertal |
16:35-17:20 | Explainable machine learning in drug discovery Jürgen Bajorath, Bonn |
17:15 – 17:20 | Closing Words (FJB) |
18:30 – 19:15 | Dortmunder U | ||
20:00 – | Dinner |
Main Organizers
Annika Schuster, Frauke Stoll, and Florian J. Boge